Climate Risk Disasters Data Understanding the Impacts
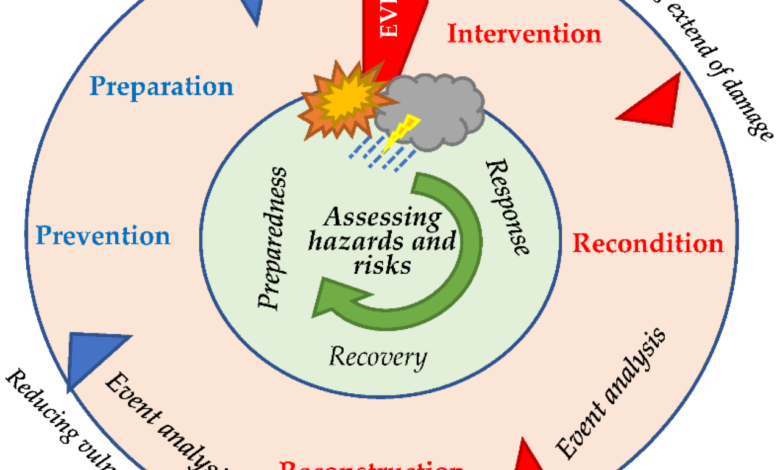
Climate risk disasters data is crucial for understanding and mitigating the impacts of climate change. This data encompasses everything from floods and droughts to storms, providing insights into the spatial, temporal, and attribute characteristics of these events. Sources range from government agencies and research institutions to private companies, each contributing valuable information to a complete picture of the challenges ahead.
Analyzing this data helps us understand the past, present, and future risks, allowing for better disaster response and proactive mitigation strategies.
The different types of disasters, like floods and droughts, have distinct characteristics. Floods are characterized by the affected area, duration, and water depth. Droughts, conversely, focus on affected regions, duration of dryness, and precipitation levels. Understanding these nuances is key to effective disaster management.
Defining Climate Risk Disasters Data
Climate risk disaster data is crucial for understanding and mitigating the impacts of increasingly frequent and intense extreme weather events. This data provides a historical record of past disasters, allowing for analysis of trends, patterns, and potential future risks. Understanding these patterns is essential for proactive disaster preparedness and effective resource allocation.This data encompasses a wide range of information related to climate-related disasters, from the precise location of flood damage to the severity of drought conditions.
Climate risk disaster data is crucial for understanding potential future threats. Knowing the impact of extreme weather events is vital, but also understanding the political landscape is equally important. For instance, the upcoming Nevada caucus primary, as explained in the Nevada caucus primary explainer , will likely influence future policy decisions, which in turn could impact how we prepare for and respond to climate-related disasters.
Ultimately, a comprehensive approach to climate risk disaster data analysis needs to consider both scientific and political factors.
It allows researchers and policymakers to develop targeted strategies for reducing vulnerability and building resilience. It is not just about documenting events, but about extracting meaningful insights to improve future outcomes.
Types of Climate-Related Disasters
Climate risk disaster data covers various types of climate-related disasters, including but not limited to floods, droughts, storms, wildfires, heatwaves, and sea-level rise. Each type of disaster presents unique challenges in terms of data collection, analysis, and response. Understanding the specific characteristics of each disaster is critical for effective mitigation strategies.
Sources of Climate Risk Disaster Data
Numerous sources contribute to the compilation of climate risk disaster data. Government agencies, such as the National Oceanic and Atmospheric Administration (NOAA) and the United States Geological Survey (USGS), play a vital role in collecting and disseminating data related to various climate-related disasters. Research institutions, with their specialized expertise, contribute to the analysis and interpretation of this data, often producing reports and models.
Private companies also collect and process relevant data, sometimes offering specialized services and products based on this information.
Characteristics and Formats of Climate Risk Disaster Data
Climate risk disaster data possesses key characteristics that define its nature and usability. The spatial aspect identifies the geographical location of the affected area. Temporal information tracks the duration and frequency of the event. Attribute data provides details such as intensity, severity, and impact on human populations and infrastructure. Data formats can range from simple tabular representations to complex geospatial datasets, requiring appropriate tools and expertise for analysis.
Comparison of Disaster Types
The following table highlights the key characteristics of flood and drought data, showcasing the diverse nature of climate risk disaster data.
Disaster Type | Data Characteristics | Example Sources |
---|---|---|
Floods | Spatial: affected areas, delineated by precise coordinates and geographic features; Temporal: duration of flooding, measured in hours, days, or weeks, with timestamps marking the onset and peak; Attribute: water depth, measured in feet or meters, and the extent of damage to property and infrastructure, measured in monetary values or building counts. | National Weather Service, USGS, local government agencies, insurance companies |
Droughts | Spatial: affected regions, identified by specific administrative boundaries or hydrological basins; Temporal: duration of dryness, measured in weeks, months, or years, with records of rainfall deficits; Attribute: precipitation levels, measured in inches or millimeters, and the impact on agriculture, water supplies, and ecosystems. | NOAA, agricultural agencies, water management authorities, satellite imagery providers |
Storms | Spatial: path of the storm, intensity, wind speed; Temporal: duration of the storm, including time of onset, peak intensity, and dissipation; Attribute: wind speed, rainfall, damage to infrastructure, casualties, and displacement of populations. | National Hurricane Center, National Weather Service, satellite imagery providers |
Wildfires | Spatial: area burned, spread of fire, affected ecosystems; Temporal: duration of the fire, intensity of the fire, including time of onset and containment; Attribute: burned area, severity of damage, including property damage, air quality, and ecological impacts. | Forest Service, local fire departments, satellite imagery providers |
Data Collection and Measurement
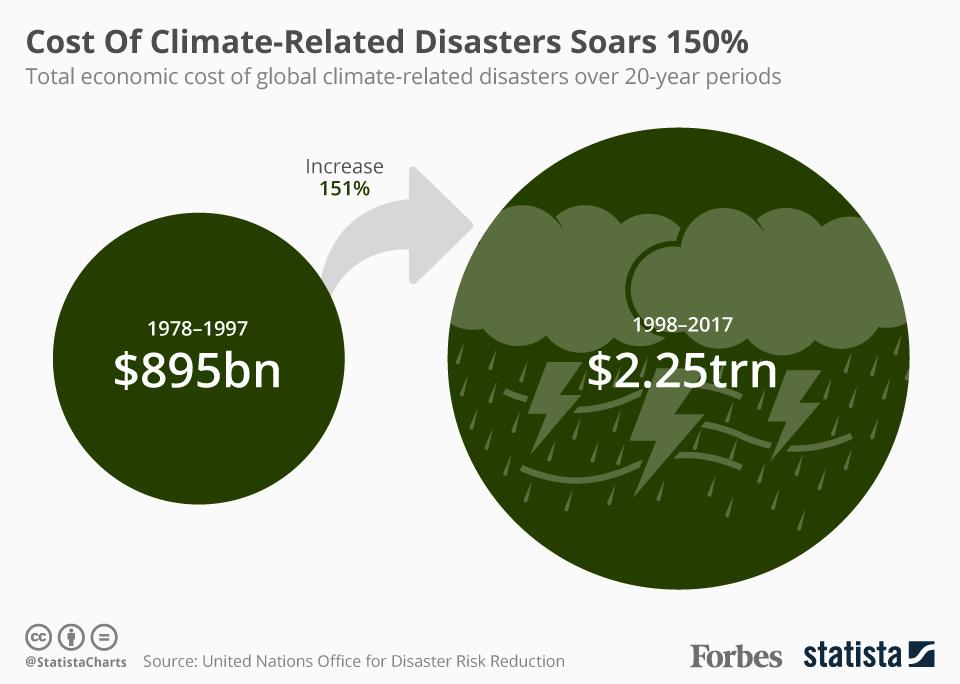
Gathering reliable data on climate risk disasters is crucial for effective response and mitigation strategies. Accurate information allows for better understanding of patterns, impacts, and vulnerabilities, enabling the development of targeted interventions. The diverse nature of these events necessitates a multifaceted approach to data collection, encompassing various methods and technologies. This process also highlights the critical need for consistent data standards across regions and time periods.Understanding the methods used to collect climate risk disaster data is fundamental to assessing the reliability and accuracy of the information.
This involves a range of techniques, from traditional ground-based observations to cutting-edge satellite technologies. Different methods offer varying strengths and weaknesses in terms of coverage, resolution, and cost. The selection of the appropriate method depends heavily on the specific disaster, the region affected, and the resources available.
Climate risk disaster data is crucial for understanding and mitigating future impacts. Knowing the patterns and frequency of extreme weather events is vital, but sometimes the data gets overshadowed by other topics, like determining the best last name for a child. The recent trends in naming conventions, for example, the apellido bebe madre padre debate, can be interesting to follow, but it’s important to remember that the data about climate risks is what will ultimately shape our future.
We need to prioritize understanding these data sets to prepare for a sustainable world.
Data Collection Methods
Various methods are used to collect climate risk disaster data, each with its own strengths and weaknesses. The choice of method often depends on the scale of the event, the geographic location, and the resources available.
Data Collection Method | Strengths | Weaknesses |
---|---|---|
Satellite imagery | Provides large-scale coverage, allowing for real-time monitoring of disaster events, such as floods, wildfires, and storms. Satellite imagery can capture extensive areas quickly, often before ground teams can arrive. | Resolution limitations can restrict the detail of the information gathered. Cloud cover can obscure views, hindering data acquisition in certain circumstances. Processing and interpretation of satellite data require specialized expertise and sophisticated software. |
Ground-based sensors | Offers high-resolution, detailed measurements of specific parameters, such as temperature, rainfall, wind speed, and soil moisture. This level of precision is crucial for understanding the local impact of disasters. Ground-based sensors offer the advantage of immediate feedback, allowing for immediate action in response to changing conditions. | Limited geographic coverage is a significant drawback. Deployment and maintenance of ground-based sensors can be expensive and logistically challenging. Data consistency across different locations can be a problem. The locations and types of sensors used are critical for accurate interpretation. |
Social media and citizen reporting | Rapidly gathers information from affected communities, providing insights into the immediate needs and challenges. Social media platforms and mobile reporting tools can provide valuable information about the extent of damage and the needs of those affected. These platforms offer an immediate response channel, particularly in areas with limited communication infrastructure. | Accuracy and reliability of information can be compromised due to potential biases and misinformation. Ensuring data verification and quality control is essential. The need for trained personnel to filter and validate the information is a considerable challenge. |
Historical records and databases | Provide valuable context and insights into past disaster events, enabling the identification of trends and patterns. Analysis of historical records can identify vulnerable areas, predict potential risks, and improve mitigation strategies. | Data may not be readily available for all areas or time periods. Data quality and accuracy may vary depending on the source and recording methods of the past. Maintaining data consistency over extended time periods can be challenging. |
Importance of Data Quality and Accuracy
Data quality and accuracy are paramount in disaster response and mitigation. Inaccurate or incomplete data can lead to misallocation of resources, ineffective response strategies, and potentially exacerbate the situation. For example, inaccurate assessments of damage from a flood can lead to insufficient rescue and relief efforts, potentially endangering lives and exacerbating hardship.
Challenges in Data Collection, Climate risk disasters data
Consistency in data collection across different regions and time periods presents significant challenges. Different regions have varying capacities for data collection and reporting. Historical data may be incomplete or inconsistent, hindering trend analysis. Standardization of data formats and methodologies is necessary to enable effective comparisons and analyses.
Innovative Data Collection Techniques
Several innovative data collection techniques are emerging, offering more comprehensive and real-time data. Drone imagery is increasingly being used for detailed assessments of damage, particularly in hard-to-reach areas. Remote sensing techniques, combining satellite imagery and ground-based sensors, offer a powerful way to monitor disaster events and assess their impacts.
Data Analysis and Interpretation: Climate Risk Disasters Data
Unraveling the complexities of climate risk disaster data requires sophisticated analytical methods to extract meaningful insights. Understanding the patterns, trends, and impacts of these events is crucial for developing effective mitigation and adaptation strategies. This process involves examining various datasets, identifying correlations, and utilizing statistical models to forecast future risks.The interpretation of this data goes beyond simple observation; it necessitates a deep dive into the factors influencing disaster vulnerability and resilience.
By analyzing historical patterns, we can identify areas most at risk and tailor interventions to strengthen communities’ capacity to withstand future events.
Analytical Techniques for Assessing Disaster Impacts
Understanding the impact of climate disasters on communities necessitates the use of diverse analytical techniques. These methods help to quantify the effects on various sectors, including infrastructure, agriculture, and human health.
- Spatial analysis: This technique uses geographic information systems (GIS) to map disaster occurrences, identify vulnerable areas, and assess the spatial extent of damage. For instance, analyzing the spatial distribution of flood events over a period of 20 years can reveal areas repeatedly affected, highlighting the need for enhanced flood defenses in those regions.
- Social vulnerability assessments: These assessments consider socioeconomic factors like poverty, access to resources, and community organization to determine the susceptibility of different populations to disasters. Analyzing demographic data alongside disaster impact data helps understand which groups are disproportionately affected and develop targeted support strategies.
- Economic impact analysis: Quantifying the economic losses from a disaster is essential for resource allocation and recovery planning. This involves assessing damage to infrastructure, agricultural production, and business disruption. For example, analyzing insurance claims data after a hurricane can reveal the economic burden of the event and the need for improved disaster insurance programs.
Statistical Modeling in Forecasting Future Risks
Statistical models are essential tools for predicting future disaster risks. They allow us to identify trends and relationships in historical data, enabling informed predictions about future occurrences.
- Regression analysis: This method investigates the relationship between disaster occurrence and various factors like temperature, rainfall, and population density. For example, regression models can show how increased temperatures correlate with an increase in the frequency of wildfires, providing insights for proactive fire prevention strategies.
- Time series analysis: This approach examines historical data to identify patterns and predict future trends in disaster occurrences. Analyzing historical earthquake data can reveal cyclical patterns, potentially enabling more accurate predictions of future events.
Machine Learning in Predicting Disaster Patterns
Machine learning algorithms are increasingly used to analyze complex disaster data and predict future patterns. Their ability to identify intricate relationships and complex patterns makes them powerful tools for forecasting.
- Predictive modeling: Machine learning models can be trained on historical data to predict the likelihood of future disasters. By considering various factors, such as weather patterns and geological conditions, models can generate probabilities of disaster events, enabling proactive preparedness and response.
- Pattern recognition: Machine learning algorithms can identify patterns and anomalies in large datasets, such as satellite imagery or social media data. This can provide early warning signals of impending disasters, like identifying unusual weather patterns or social media discussions indicating a potential event.
Steps in a Disaster Risk Assessment
A structured approach to disaster risk assessment is critical for effective mitigation and adaptation strategies. The following table Artikels the key steps involved in the process.
Step | Description |
---|---|
1. Data Collection | Gather data on past disasters, including location, intensity, and impact on communities. |
2. Data Analysis | Analyze the collected data to identify patterns, trends, and correlations between different variables. |
3. Vulnerability Assessment | Identify vulnerable populations and infrastructure based on socioeconomic factors and geographic location. |
4. Risk Modeling | Develop models to project future disaster risks and potential impacts. |
5. Risk Communication | Communicate findings to stakeholders to inform decision-making and implement mitigation strategies. |
Data Visualization and Communication
Climate risk disaster data, when effectively visualized and communicated, becomes a powerful tool for understanding, mitigating, and responding to these events. Clear and accessible representations of this data are crucial for informing policy decisions, public awareness campaigns, and ultimately, saving lives and reducing societal impact. The ability to transform complex datasets into easily digestible visuals allows stakeholders to identify trends, understand vulnerabilities, and plan for future events.Understanding the diverse needs of different audiences is paramount in communicating climate risk disaster data effectively.
Policymakers require data-driven insights to formulate strategic responses and allocate resources. The public needs easily understandable information to prepare for potential hazards and contribute to disaster preparedness. Visualizations that cater to these differing needs can foster a more collaborative and effective approach to tackling climate risks.
Effective Visualization Methods
Visualizing climate risk disaster data requires a multifaceted approach. Choosing the right visualization technique is essential for conveying the intended message accurately and compellingly. Choropleth maps, for instance, are well-suited for displaying spatial patterns of disaster impacts, highlighting areas with higher vulnerability or damage levels. These maps can effectively illustrate the geographic distribution of affected populations and infrastructure, which can be invaluable for resource allocation and targeted relief efforts.
Interactive maps, capable of filtering and zooming, provide even more detailed insights. By allowing users to explore data at varying levels of granularity, these interactive maps empower users to understand specific areas of concern.
Climate risk disaster data is crucial for understanding potential threats, but it’s also vital to consider the broader ethical implications. For example, when considering the purchase of historical documents, like letters from strangers, the ethics of acquiring such items becomes important. Examining the ethical considerations surrounding the purchase of historical artifacts like those discussed in stranger letters purchase ethics provides a fascinating parallel.
Ultimately, understanding the ethical implications of accessing and using climate risk disaster data is just as important as the data itself.
Communication Strategies for Different Audiences
Effective communication is critical for disseminating climate risk disaster data to various stakeholders. Policymakers benefit from concise summaries and data-driven analyses that highlight the economic and social costs of inaction. The public, conversely, requires more accessible information presented in clear, easily digestible formats. This may include infographics, animated videos, or simple text summaries. The use of clear and accessible language, avoiding jargon, is paramount to ensure the message is conveyed effectively to all audiences.
Climate risk disaster data is crucial for understanding global vulnerabilities. While the current geopolitical situation, like the ongoing Israel-Hamas hostages ceasefire talks here , dominates headlines, we shouldn’t lose sight of the long-term risks. Analyzing this data helps us prepare for and mitigate future disasters, no matter the immediate global news cycles.
Examples of clear and concise communication include providing specific examples of how past disasters have impacted similar communities, which can foster empathy and understanding.
Interactive Maps for Disaster Data
Interactive maps provide an invaluable tool for visualizing climate risk disaster data. These maps allow users to explore spatial patterns, drill down into specific locations, and filter data based on various criteria. For instance, a map displaying historical flood events could allow users to identify areas most prone to flooding based on rainfall patterns, elevation, and past flood records.
Such maps can also highlight infrastructure vulnerable to flooding, such as hospitals, schools, and critical lifelines. This allows for a more thorough understanding of the risks faced by specific communities and empowers stakeholders to implement preventive measures.
Developing Dashboards for Disaster Trend Tracking
Dashboards are powerful tools for tracking disaster trends over time. By consolidating key metrics and visualizations, dashboards provide a comprehensive overview of disaster activity. A dashboard could integrate data on flood frequency, hurricane intensity, and wildfire outbreaks to reveal patterns and potential long-term trends. This aggregated data can then be used to refine risk assessments and predict future disaster scenarios.
Dashboards can also incorporate predictive models to anticipate potential disaster impacts and offer proactive response strategies.
Visualization Tools and Use Cases
Visualization Tool | Data Type | Use Case |
---|---|---|
Choropleth maps | Spatial data with associated values | Show distribution of disaster impacts across regions |
Time series plots | Temporal data | Track changes in disaster frequency over time |
Heatmaps | Spatial data with intensity values | Visualize the intensity of disaster impacts across a region |
Network graphs | Data representing relationships between entities | Illustrate the interconnectedness of infrastructure and communities affected by a disaster |
Scatter plots | Data with two variables | Analyze the correlation between variables like rainfall and flood damage |
Data Management and Accessibility
Managing climate risk disaster data effectively is crucial for informed decision-making and proactive mitigation strategies. Proper storage, accessibility, and interoperability are essential to leverage the insights embedded within this data. This crucial step allows researchers, policymakers, and communities to understand trends, predict future risks, and develop effective responses. Without proper management, valuable information can be lost or rendered unusable, hindering progress in addressing climate change impacts.
Best Practices for Storing and Managing Climate Risk Disaster Data
Robust data management practices are paramount for ensuring the long-term usability and value of climate risk disaster data. These practices encompass secure storage methods, version control, and comprehensive metadata descriptions. Data should be organized in a structured format, ideally using a relational database or a specialized data management system. Regular backups and disaster recovery plans are critical to prevent data loss.
Metadata should be detailed and include information about the data’s source, collection method, time period, location, and any relevant contextual information.
Importance of Data Interoperability and Standardization
Data interoperability is critical for seamless data exchange and analysis. Standardized formats, such as those provided by organizations like the Open Geospatial Consortium (OGC), allow different datasets to be combined and analyzed. This ensures compatibility across various platforms and tools. A lack of standardization creates difficulties in aggregating information from multiple sources, hindering comprehensive risk assessments. Standardization also helps to avoid discrepancies and errors, resulting in more accurate and reliable insights.
Role of Open Data Initiatives in Facilitating Access
Open data initiatives play a vital role in democratizing access to climate risk disaster data. Making this data freely available promotes collaboration, innovation, and transparency. Open data licenses allow researchers, policymakers, and the public to utilize and share the data, enabling them to develop tailored solutions for their specific needs. Open data portals and repositories make data discoverable and accessible to a wider audience.
Climate risk disaster data is crucial for understanding and mitigating the impacts of extreme weather events. It’s fascinating how the recent news about Arthur Smith being hired as the Steelers offensive coordinator, arthur smith hired steelers offensive coordinator , might influence future strategies for managing these risks, particularly as related to sports facility planning in vulnerable areas.
Ultimately, this data informs crucial decisions for long-term resilience.
Data Repositories Hosting Climate Risk Disaster Data
Several repositories host climate risk disaster data. Some notable examples include:
- The National Oceanic and Atmospheric Administration (NOAA) archives various datasets related to weather patterns, storm events, and climate change impacts.
- The United Nations Environment Programme (UNEP) provides data on environmental hazards, including climate-related disasters.
- The European Union’s Copernicus program gathers and disseminates satellite imagery and data related to environmental monitoring, including climate-related disasters.
- Various national meteorological agencies maintain records of weather events and climate data.
Structuring Data to Maximize Searchability
Effective data structuring is essential for facilitating efficient searching and retrieval of climate risk disaster information. The structure should allow for easy identification and filtering of data based on various criteria. Using a well-defined taxonomy, including standardized terms and concepts, is crucial. This taxonomy should allow users to easily locate data relevant to specific regions, time periods, or disaster types.
Thorough documentation of the data, including clear descriptions and metadata, helps users understand the context and meaning of the data. This comprehensive documentation also assists in the effective search and interpretation of data.
Final Thoughts
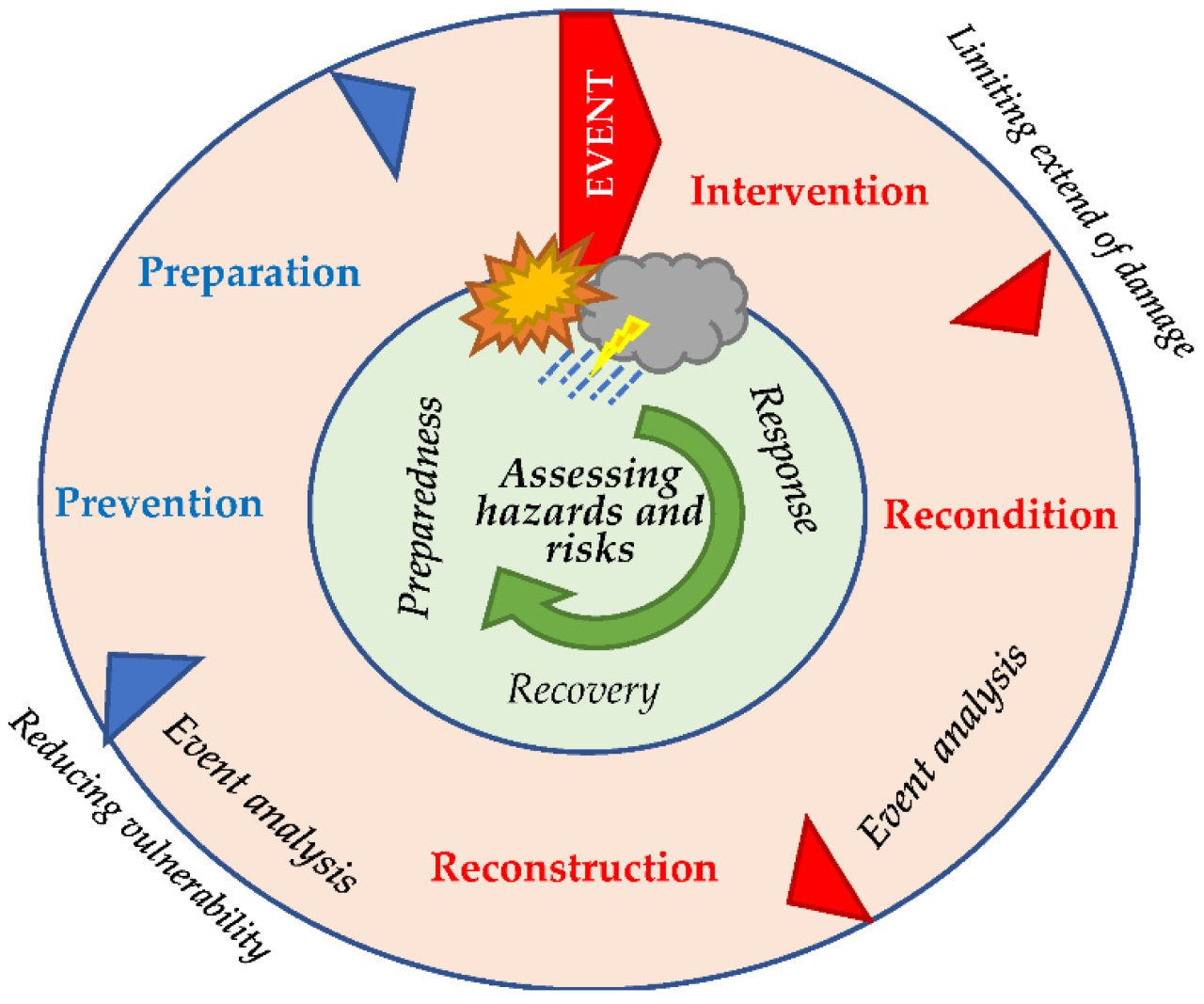
In conclusion, climate risk disasters data offers a comprehensive view of the impacts of climate change. By understanding the sources, characteristics, collection methods, and analysis techniques, we can develop effective strategies to mitigate future risks. Visualization and communication are critical to conveying this information to policymakers and the public, ultimately enabling proactive measures and better disaster preparedness.
FAQ
What are some limitations in collecting climate risk disaster data?
Collecting consistent data across regions and time periods presents challenges. Different regions might use varying methodologies or have gaps in data collection, and maintaining historical records for long periods can be difficult and costly. Also, some data sources might be more readily available in certain regions than others, leading to an uneven dataset.
How can machine learning be used in predicting disaster patterns?
Machine learning algorithms can analyze vast datasets of historical disaster data to identify patterns and predict future occurrences. This can help with early warning systems and resource allocation, allowing for better response times and minimizing damage.
What are the best practices for storing and managing this data?
Best practices for data storage and management involve data interoperability and standardization, using robust systems to ensure long-term accessibility. Proper metadata management is crucial for efficient retrieval and analysis. Data should be stored securely and redundantly to avoid data loss.
How can data visualization tools help communicate disaster risk?
Interactive maps and dashboards can visually represent the spatial and temporal patterns of disaster risk. These tools allow for easy understanding of the impact across different communities and regions, which is vital for effective communication with stakeholders and the public.