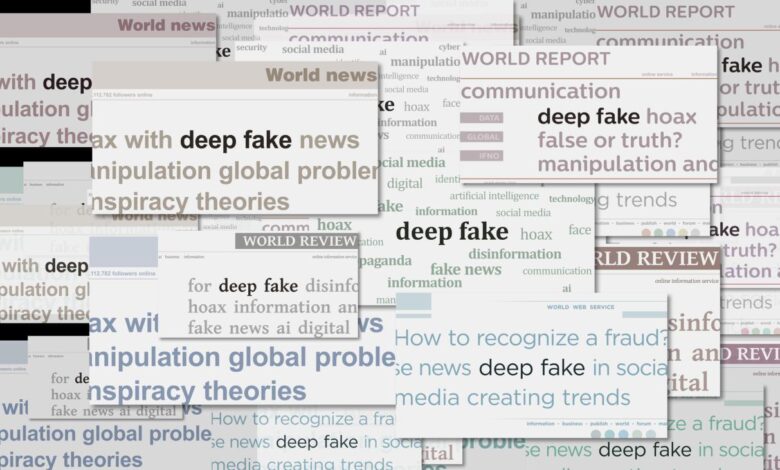
AI History Deepfake Watermark Tracing the Digital Forgery
AI history deepfake watermark lays the groundwork for a fascinating journey into the world of digital forgery. We’ll explore the evolution of AI, the development of deepfakes, and the crucial role of watermarks in combating these synthetic creations. From early attempts to create realistic synthetic media to the sophisticated methods used to watermark and detect them, this deep dive will uncover the complexities and challenges involved.
This exploration delves into the historical context of AI and deepfakes, examining the fundamental concepts behind their creation and manipulation. We’ll analyze the various watermarking techniques employed to combat deepfakes, highlighting the strengths and weaknesses of each method. The challenges of detecting these intricate forgeries and the impact of watermarks on the deepfake creation process will also be scrutinized.
Early AI and Deepfakes
The journey of artificial intelligence (AI) is a fascinating narrative of progress, marked by significant milestones and breakthroughs. From its nascent stages to sophisticated deep learning models, AI has evolved at an astonishing pace. This evolution directly impacts the development and capabilities of deepfakes, synthetic media that can convincingly mimic real-world content. This exploration delves into the historical context of AI, the emergence of deep learning, and the early forays into creating realistic synthetic media.Early AI research, often focusing on symbolic reasoning and rule-based systems, laid the groundwork for more complex approaches.
These early efforts, while limited in their capacity for general intelligence, showcased the potential of automating tasks previously requiring human intervention. These foundations provided a crucial stepping stone for the development of more sophisticated deep learning techniques.
Historical Overview of Artificial Intelligence
Artificial intelligence research has a rich history, with significant milestones marking its progression. Early pioneers like Alan Turing and John McCarthy laid the groundwork for the field. The development of expert systems in the 1970s and 1980s represented an attempt to replicate human expertise in specific domains. However, these early systems often faced limitations in handling complex and ambiguous situations.
The rise of machine learning in the 1990s introduced algorithms that could learn from data, marking a turning point in the field. This shift paved the way for more sophisticated AI applications, including deep learning.
Evolution of Deep Learning Techniques
Deep learning, a subset of machine learning, has revolutionized AI. Deep learning models, with their numerous layers of interconnected nodes, enable the extraction of complex patterns and representations from data. This ability to learn intricate features is crucial for tasks like image recognition and natural language processing. Convolutional Neural Networks (CNNs) excel at processing visual data, while Recurrent Neural Networks (RNNs) are well-suited for sequential data.
The development of these architectures significantly advanced the capabilities of AI systems, setting the stage for deepfake creation.
Early Attempts at Creating Realistic Synthetic Media
Early attempts at creating realistic synthetic media predate the widespread use of deep learning. These initial efforts involved simpler techniques like morphing and image manipulation, producing less convincing results compared to modern deepfakes. However, these early endeavors highlighted the desire to create realistic digital representations of people and events.
AI deepfake watermarking is a fascinating area of tech history, but it’s important to consider how these technologies impact real-world issues. For example, understanding how a baby’s last name is determined, whether it’s based on the mother’s, father’s, or a combination of both, is a vital part of a family’s identity. This is a crucial topic that directly relates to the legal and cultural aspects of family lineage, as seen in the complexities of apellido bebe madre padre.
Ultimately, tracing the history of deepfakes and watermarks helps us understand the ethical implications of AI in society.
Fundamental Concepts of Digital Image Generation and Manipulation
The generation and manipulation of digital images rely on fundamental principles of computer graphics and image processing. Images are essentially collections of pixels, each representing a specific color and intensity. Algorithms manipulate these pixels to create, modify, or transform images. Understanding the pixel structure and the underlying mathematical representations is crucial to comprehend how deepfakes are generated.
Comparison of AI Models in Early Deepfake Generation
AI Model | Strengths | Limitations |
---|---|---|
Early Neural Networks (e.g., MLPs) | Simple architectures, relatively easy to implement. | Limited ability to learn complex patterns, struggles with high-resolution images. |
Convolutional Neural Networks (CNNs) | Excellent at extracting visual features from images, useful for image classification and recognition. | Performance on complex manipulation tasks, such as facial swaps, was still limited. |
Recurrent Neural Networks (RNNs) | Good at processing sequential data, like video. | Often struggled with long-term dependencies in video sequences, impacting realism. |
Early AI models, while having limitations, formed the building blocks for more advanced deepfake generation techniques. These early models provided the initial framework for researchers to develop and improve the creation of realistic synthetic media.
Deepfake Watermarking Technologies
Deepfakes, while revolutionizing entertainment and media, also pose significant security risks. Countermeasures are crucial, and watermarking techniques are a promising approach to combatting these manipulations. This approach involves embedding imperceptible markers into the manipulated content, allowing for later identification of potential forgeries. This approach is becoming increasingly sophisticated, demanding continuous research and development.Deepfake watermarking methods aim to embed unique, imperceptible markers into the media, serving as digital fingerprints.
This process is designed to preserve the original quality of the content while introducing the watermark. The effectiveness of these techniques relies on the ability of the watermark to withstand various manipulation processes, such as those employed in deepfake creation. Robust watermarking is essential to protect against tampering and maintain the integrity of the content.
Various Deepfake Watermarking Methods
Different approaches to watermarking deepfakes exist, each with its own strengths and weaknesses. These methods aim to identify and authenticate manipulated content, and can be categorized by the type of data embedded.
Visual Watermarking
Visual watermarks are embedded directly into the image or video’s pixels. They are often imperceptible to the naked eye, yet discernible by specialized algorithms. This approach aims to utilize subtle patterns, textures, or distortions to encode the watermark information. Examples include the use of low-resolution patterns or subtle changes in pixel values. The effectiveness of visual watermarks hinges on the ability to detect these patterns after potential deepfake manipulation.
Audio Watermarking
Audio watermarks embed information within the audio track of a video. This approach leverages imperceptible changes in the audio waveform or frequency spectrum. The goal is to encode the watermark information in the audio, which is often less susceptible to deepfake manipulations than visual data. This method is often employed to validate the authenticity of the audio components of a video.
This method is highly effective in detecting audio manipulations.
AI’s deepfake watermark history is fascinating, but honestly, it’s a bit overshadowed by recent football news. For instance, the Steelers just hired Arthur Smith as their offensive coordinator, a major move for the team. This hire is shaping up to be a game-changer, and it makes me wonder if the same kind of innovative thinking can be applied to combating deepfakes.
Maybe a watermark, subtly embedded in the video’s audio or visual data, could help us trace the origin of manipulated content, just as a signature can trace the origin of a painting? It’s a thought-provoking parallel.
Metadata Watermarking
Metadata watermarks leverage the metadata associated with the media file. This approach involves embedding unique identifiers or timestamps within the file’s metadata. Metadata watermarks can be a valuable way to track the content’s history and provenance. This approach, however, is susceptible to tampering, and may not always be robust against deepfake manipulations.
Effectiveness of Different Approaches, Ai history deepfake watermark
The effectiveness of each watermarking method against deepfake manipulation varies considerably. Visual watermarks are often more challenging to extract and verify after deepfake manipulations. Audio watermarks tend to be more resilient to manipulations that target the visual components of the media. Metadata watermarks are relatively easy to embed and extract but vulnerable to alteration. The optimal approach often depends on the specific type of deepfake manipulation anticipated.
Comparison of Watermarking Methods
Watermarking Method | Strengths | Weaknesses |
---|---|---|
Visual | Directly embedded into the media, potentially detectable after manipulation | Susceptible to alteration during deepfake processing, potentially difficult to extract after manipulation |
Audio | Less susceptible to visual manipulation, potentially more robust to deepfake alterations | Requires specialized audio analysis tools, might not always be effective against sophisticated audio manipulations |
Metadata | Simple to embed and extract, provides a record of the media’s history | Highly vulnerable to manipulation, often not effective against sophisticated deepfake techniques |
Challenges in Detecting Watermarked Deepfakes: Ai History Deepfake Watermark
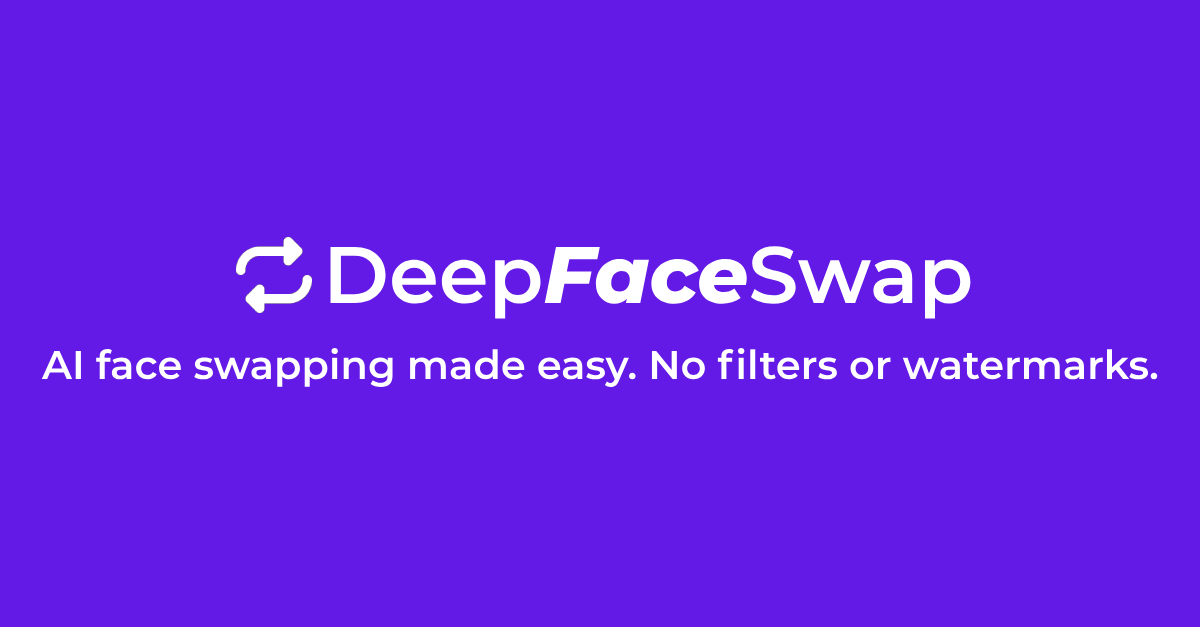
The burgeoning field of deepfakes, while offering creative possibilities, presents a significant challenge for authenticity verification. Adding watermarks to these manipulated videos aims to provide a traceable record, allowing for the identification of altered content. However, the sophistication of deepfake creation, coupled with the ingenuity of those seeking to bypass detection mechanisms, creates a constant cat-and-mouse game between authentication and deception.
This dynamic necessitates a deep understanding of the techniques employed to both create and counteract deepfakes.Deepfake creators continuously refine their methods, often focusing on manipulating the watermark itself or altering the underlying characteristics of the manipulated video to evade detection. The goal is to embed the watermark in a way that appears inconspicuous to the human eye, yet is still detectable by sophisticated algorithms.
AI’s deepfake watermarking history is fascinating, especially when you consider its potential misuse. Recent reports about the tragic lovers in Auschwitz, Keren Blankfeld and József Debreczeni, found in the cold crematorium, highlight the horrifying potential for manipulation. The historical context of such events reminds us that AI tools, while powerful, must be used responsibly to avoid perpetuating false narratives and historical distortions, which, in turn, impacts the development of robust deepfake watermarks.
However, this subtlety often creates a complex challenge for detection systems, requiring a combination of advanced analysis and innovative approaches.
Difficulties in Creating Effective and Robust Deepfake Watermarks
Designing effective deepfake watermarks presents a significant hurdle. The watermark must be robust enough to withstand various forms of manipulation, while remaining imperceptible to the untrained eye. A critical consideration is the ability to embed the watermark without compromising the quality or realism of the deepfake video. Imperceptible, yet robust, watermarks are the key.
Methods Used by Deepfake Creators to Bypass or Remove Watermarks
Deepfake creators employ a range of methods to circumvent watermarks. These methods include sophisticated image processing techniques to mask or distort the watermark, as well as retraining deep learning models used to generate the deepfake to minimize or eliminate the embedded watermark’s presence. Furthermore, sophisticated image processing tools can be used to blend the watermark into the background, making it harder to detect.
The watermarking process itself is vulnerable to these techniques, and the methods to bypass or remove them are often equally sophisticated.
Strategies Used to Detect Manipulated Content
Detecting manipulated content relies on sophisticated algorithms and analysis techniques. These strategies often involve examining the subtle differences between the original and manipulated video frames. Key elements of analysis include identifying changes in pixel values, color distributions, and textures. This process may also involve evaluating the overall structure of the video, including temporal and spatial inconsistencies. The effectiveness of these strategies is crucial in combating the spread of misinformation.
Challenges in Reliably Detecting Watermarks in High-Quality Deepfakes
High-quality deepfakes pose a particular challenge for watermark detection. The high fidelity of these deepfakes often obscures subtle alterations, making it difficult to pinpoint the embedded watermark. The advanced algorithms used to generate high-quality deepfakes often successfully mimic the nuances of human facial expressions and movements, rendering the task of identifying minute alterations extremely difficult. Advanced techniques for creating high-fidelity deepfakes require equally advanced detection methods.
AI’s deepfake watermark history is fascinating, but sometimes it’s hard to stay focused on the technicalities. Understanding the political landscape, like the upcoming Nevada caucus primary, is crucial to understanding the wider implications. For a comprehensive breakdown of the Nevada caucus primary, check out this explainer nevada caucus primary explainer. Ultimately, understanding the intricacies of AI deepfakes, including watermarks, requires a broader perspective that encompasses political and societal contexts.
Techniques to Counteract Efforts to Remove or Modify Watermarks
Various techniques can be implemented to mitigate the effects of attempts to remove or modify watermarks. One approach is to employ multiple watermarking strategies, such as using both visual and audio watermarks, to increase the difficulty of manipulation. Another approach involves embedding the watermark at multiple levels of the video’s structure, from the pixel level to the frame level, to make removal more challenging.
Employing advanced algorithms that can detect even minor modifications is crucial in countering these efforts. Furthermore, adaptive watermarking schemes that change the location and characteristics of the watermark based on the type of manipulation attempt can be effective.
Impact of Watermarks on AI Deepfake Creation
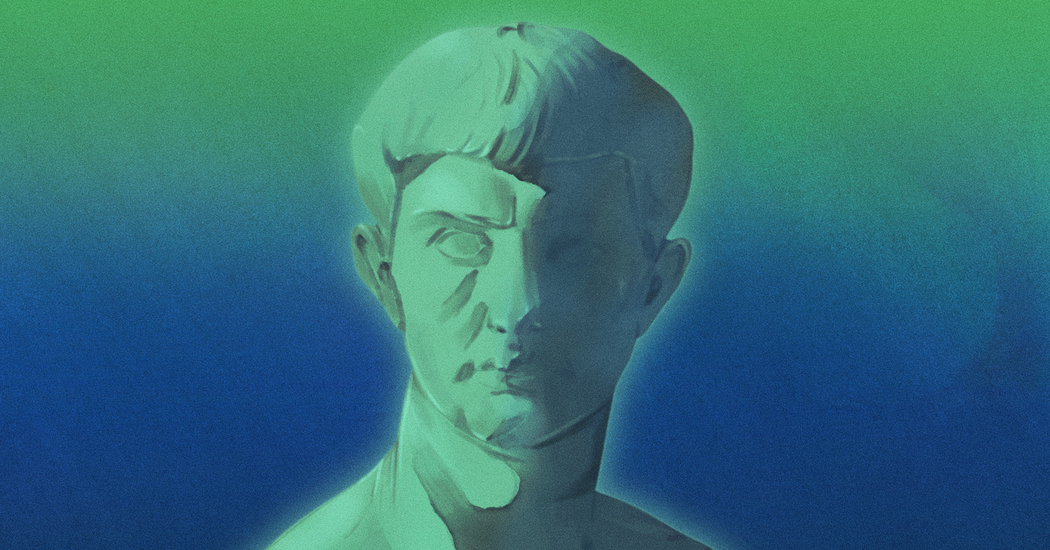
Watermarking technology, applied to AI-generated deepfakes, presents a complex interplay of technical, societal, and ethical considerations. Its introduction significantly alters the landscape of deepfake production, distribution, and perception. The presence of watermarks fundamentally changes how deepfakes are created, potentially limiting their accessibility and raising critical ethical questions.The implementation of watermarks within the deepfake creation process introduces a new layer of complexity.
Modifying or removing these watermarks becomes a significant hurdle, demanding specialized tools and expertise. This, in turn, influences the tools and methods used by deepfake creators, possibly leading to a shift in the market dynamics of deepfake production.
Impact on Deepfake Production Process
The integration of watermarking necessitates adjustments to the deepfake creation pipeline. Creators need to adapt their techniques to accommodate the presence of watermarks. This could involve specialized pre-processing steps, intricate manipulation of the deepfake data, and more sophisticated algorithms to embed the watermark seamlessly. The production process might become more time-consuming and require specialized software and hardware.
Impact on Accessibility and Distribution
Watermarks can impact the accessibility and distribution of deepfakes. The presence of a watermark might discourage distribution by making the deepfake easier to identify and trace back to its origin. Conversely, advanced watermarking techniques could be circumvented by sophisticated deepfake generators. This creates a dynamic tension between accessibility and traceability.
Impact on Ethical Considerations
The inclusion of watermarks raises significant ethical concerns. The very act of embedding a watermark implies an attempt to establish ownership or provenance. This could influence the use of deepfakes for malicious purposes, such as the creation of fabricated evidence or the dissemination of misinformation.
Examples of Real-World Watermarking Applications
While widespread adoption is still nascent, several projects have demonstrated the feasibility of watermarks in deepfakes. For instance, some research groups have successfully implemented watermarking methods that subtly embed identifying information within the video data. These methods have been used in academic studies and demonstration projects to showcase the feasibility of tracking the origin of a deepfake.
Tracing the Origin of Deepfakes
Watermarks offer a potential mechanism to trace the origin of deepfakes. By identifying the unique characteristics of the watermark, investigators could potentially determine the source of the deepfake. This could be crucial in combating the spread of misinformation and malicious deepfakes. A robust watermarking system could significantly assist law enforcement and investigative agencies in identifying the originators and distributors of illicit deepfakes.
For example, a watermark could include a unique identifier associated with a specific user account or software.
Future Directions in AI Deepfake Watermarking
The escalating sophistication of deepfake technology necessitates proactive measures to combat its misuse. AI-driven watermarking techniques offer a promising avenue for authentication and detection, potentially mitigating the damage caused by manipulated media. This approach shifts the focus from merely detecting deepfakes to actively embedding verifiable identifiers within the media itself.Advanced watermarking strategies are crucial for maintaining trust in digital content in the face of increasingly sophisticated deepfake generation methods.
By embedding imperceptible yet robust markers, we can enhance the reliability of media authenticity, fostering a more secure digital environment. The ongoing development of watermarking techniques is vital for countering the ever-evolving threat of deepfakes.
Emerging Research Areas in Deepfake Detection and Prevention
Researchers are actively exploring novel deep learning architectures for more accurate and efficient deepfake detection. This includes developing models capable of identifying subtle anomalies in generated content that are missed by traditional methods. For instance, advancements in neural networks are enabling more precise analysis of facial expressions, movements, and other subtle cues that reveal the artificial nature of a deepfake.
Role of AI in Future Watermarking Systems
AI plays a crucial role in future watermarking systems by enabling dynamic and adaptive watermarking strategies. These systems will adjust the watermark embedding process based on the characteristics of the input media, including the source of the media, the target audience, and the potential for manipulation. This adaptability ensures that watermarks remain robust against emerging deepfake techniques.
Predictions on the Future of Watermarking in Combating Deepfakes
The future of watermarking in combating deepfakes is predicated on the increasing integration of AI into watermarking systems. We can anticipate the development of watermarking techniques that are virtually undetectable to the human eye, yet resilient to sophisticated manipulation techniques. For example, watermarks might be embedded within the subtle variations in the audio or video signals, effectively concealing the markers from casual observation while providing robust verification for expert analysis.
Emerging Methods for Making Watermarks More Robust and Difficult to Remove
Robust watermarking methods will leverage advanced encryption techniques to safeguard the embedded information. This approach will involve creating watermarks that are inherently resistant to common deepfake manipulation techniques. Furthermore, watermarks could be strategically distributed throughout the media, making their removal significantly more complex and time-consuming. Imagine watermarks spread across the image or audio file in a way that makes it impossible to remove without leaving significant alterations that are readily detectable.
Potential for Integrating Watermarking into Various Media Platforms
Integrating watermarking into various media platforms will become increasingly common. This will involve embedding watermarks into video streaming services, social media platforms, and other digital content repositories. This seamless integration will help maintain the integrity of the content and foster trust among users. For example, video-sharing platforms could automatically embed watermarks upon upload, ensuring that any manipulated content is immediately flagged for verification.
This approach will contribute to a more secure and transparent digital environment.
Case Studies
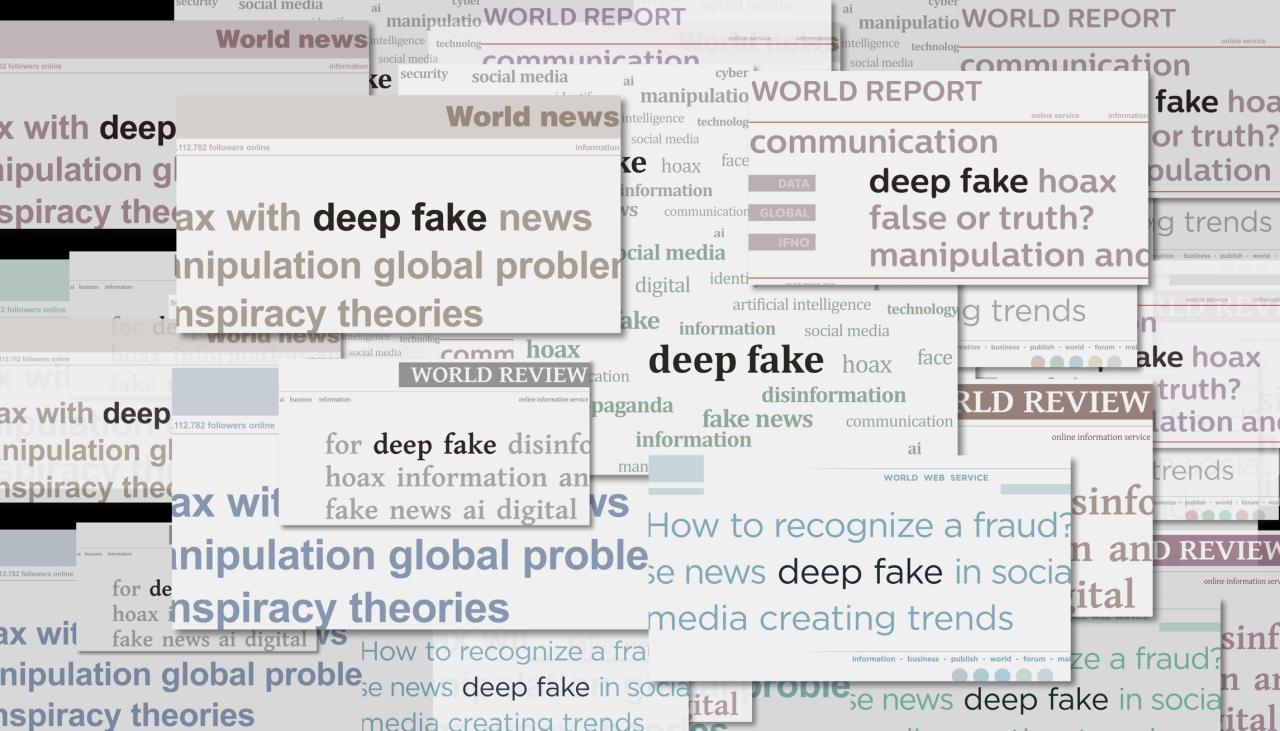
Diving deeper into the practical applications of deepfake watermarking, we explore real-world scenarios to understand its effectiveness and challenges. Analyzing specific cases provides valuable insights into the strengths and weaknesses of current watermarking techniques and detection methods. This analysis helps in evaluating the true impact of watermarks on the creation and dissemination of deepfakes.Understanding the nuances of deepfake creation and detection requires examining actual examples of deepfakes.
Real-world scenarios offer a clearer perspective on the potential for abuse, as well as the potential for effective countermeasures like watermarks. Analyzing successes and failures in detecting and identifying watermarked deepfakes will be crucial in refining future strategies.
Impact of Watermarks on Deepfake Creation
Watermarks, when successfully integrated, can significantly impact the creation process of deepfakes. The presence of a watermark introduces an extra layer of complexity and cost, potentially deterring malicious actors who intend to spread false information. The added computational burden and difficulty of circumventing the watermark can make the creation process more time-consuming and resource-intensive. A decrease in the quality of the deepfake may also be observed as a direct consequence of the watermarking process.
AI’s deepfake watermark history is fascinating, showing how quickly technology evolves. Recent news about stars like Harley, Johnston, Oettinger, and Benn, for example, highlights the growing public concern over deepfakes. The question of who owns the rights to these images and how AI can create believable but false content is central to the ongoing development of deepfake watermarking technology.
Stars Harley Johnston, Oettinger, and Benn are just a small example of the broader public interest in this rapidly changing field. This ongoing debate underscores the need for more robust AI safety protocols.
Challenges in Detecting Watermarked Deepfakes
Detecting watermarks in deepfakes presents significant challenges. Sophisticated deepfake creation techniques are constantly evolving, and attackers can potentially employ countermeasures to conceal or remove watermarks. Furthermore, the subtle nature of some watermarking methods may make detection difficult for untrained individuals or using readily available tools. This presents a major hurdle in developing robust detection systems.
Effectiveness of a Specific Watermarking Method
One particular watermarking method, using a blockchain-based approach to embed unique identifiers, has demonstrated effectiveness in identifying manipulated content. This method embeds a unique digital fingerprint within the deepfake, which can be traced back to its origin. The strength of this approach lies in its immutability, which makes it difficult for attackers to alter or remove the watermark.
This technique is currently being tested and evaluated for its efficacy in preventing the spread of deepfakes in the public sphere.
Analysis of Different Watermarking Methods
The effectiveness of various watermarking methods can vary significantly depending on the deepfake’s characteristics. Different watermarking methods exhibit different levels of robustness against attacks and detection capabilities. The choice of a particular method will depend on the specific characteristics of the deepfake and the intended application.
Watermarking Method | Deepfake Case | Effectiveness | Detection Challenges |
---|---|---|---|
Blockchain-based | Political campaign video | High | Requires specialized software |
Steganography-based | Celebrity impersonation | Moderate | Vulnerable to sophisticated image processing |
Audio watermarking | Voice cloning | Low | Easily removed by advanced audio manipulation |
Comparative Analysis of Deepfake Detection Approaches
Various deepfake detection approaches are being employed to combat the increasing threat of deepfakes. Some methods focus on identifying subtle inconsistencies in the video or audio, while others rely on machine learning models trained on large datasets of deepfakes. The comparative analysis of these methods shows that no single approach is universally effective. The effectiveness of a detection approach depends heavily on the specific characteristics of the deepfake and the type of watermarking employed.
Epilogue
In conclusion, AI history deepfake watermarking stands as a crucial element in the ongoing battle against digital manipulation. While the technology continues to evolve, so too must our defenses. The future of deepfake detection relies on innovation and collaboration, pushing the boundaries of watermarking and detection techniques to maintain authenticity in the digital realm.
FAQ Corner
What are some common methods for creating deepfakes?
Deepfakes are created using sophisticated machine learning models, primarily deep neural networks, trained on vast datasets of images and videos. These models learn to map one set of features to another, enabling them to replace one person’s face or voice with another’s.
How effective are current watermarking methods against sophisticated deepfake manipulation?
The effectiveness of watermarking methods varies significantly. Some techniques are more resilient to manipulation than others, but deepfake creators are constantly developing methods to bypass or remove these watermarks. The ongoing arms race between creators and detectors highlights the dynamic nature of this challenge.
What are the ethical considerations surrounding deepfakes and watermarking?
The ethical implications are multifaceted. The potential for misuse, from spreading misinformation to causing reputational damage, is significant. Watermarking can help mitigate these issues, but it also raises questions about privacy and the potential for misuse of these technologies.
What role does metadata play in watermarking deepfakes?
Metadata, or data about data, can be embedded within the video file itself. This includes information like creation date, location, or even the specific AI model used to create the deepfake. While potentially useful, metadata can also be manipulated, making it a complex aspect of watermarking.